Reducing operational costs and increasing operational efficiency has always been in the minds of business executives to stay ahead of ever-growing competition. The maintenance and upkeep of capital assets is a big component of operational costs. Unplanned outages caused by improper maintenance strategies or schedules are a huge drain on operational efficiencies; they lead to higher maintenance costs and lost revenue opportunities, thus hitting both the top and the bottom line. Research has shown that unplanned asset outages cost manufacturers more than $50B annually, and that the most successful manufacturing companies spend only about 25% as much on asset maintenance as the less successful ones.
While the manufacturing vertical comes to mind first when we think of assets and their maintenance, but the truth is that practically all industry verticals that depend on any form of machinery, whether it is energy with assets for power generation, oil & gas with drilling and pumping equipment, healthcare with big scanners and health monitoring equipment or agriculture, with their harvesters and tillers, they all have assets that their businesses heavily depend on. It is no surprise then, that COO organizations have been trying to move away from traditional reactive maintenance models towards condition based maintenance strategies, which are a stepping stone and fundamental prerequisite for an even better predictive asset maintenance strategy.
Challenges with Condition Monitoring
As organizations move from reactive to planned to proactive to predictive asset maintenance, they have to, at the very least, enable frequent assessment of their asset’s condition. This is exactly where organizations deploy operators to assess the condition of the assets based on the sensed values controlled by PLCs and rendered on different Human Machine Interfaces (HMI) spread across their operations.
However, to move further along in the asset maintenance efficiency journey, this ongoing assessment of the asset condition has to be automated, so slight deviation from the expected asset condition can be detected quickly and automatically. Continuous and automated monitoring of various parameters of an asset’s health becomes especially important and imperative if one wishes to go from reactive or planned or proactive asset maintenance. To add to the complexity, all of this has to be done in real-time, not just monitoring the critical health parameters of the assets, but also analyzing and detecting the deviations from the ideal ranges of these health parameters.
So, while real-time monitoring, analysis and detection of asset health parameters can help us with proactive and predictive maintenance of an asset, the shop floor or an oil rig or a power generation plant has a number of these assets that make up the operational process and often times the ideal, healthy range of an asset parameter is not an isolated value. It is, rather, contingent upon and dependent on what else is going on with other assets in that operational ecosystem. And this problem is compounded when we look at different asset classes and several assets within each asset class, all spread over a wider region or across geographies.
To truly achieve a level of maturity and advancement in asset maintenance, we are now looking at contextualization of information and data streaming from different asset classes and types, combined with interoperability across information standards, communication protocols and technical specifications.
Sensital’s iBOTics Platform for Condition Monitoring
Applications of IoT in condition monitoring are obvious and available as well. But a truly impactful and enabling solution must address the complexities that come with an operational enterprise – put it all together for all the different asset types within the enterprise.
Sensital’s iBOTics platform does exactly that and more. Not only does Sensital easily configure individual assets for monitoring its relevant parameters, but it can also model all the information flow between different assets, contextualize and triangulate information flow at any point within the operational information flow.
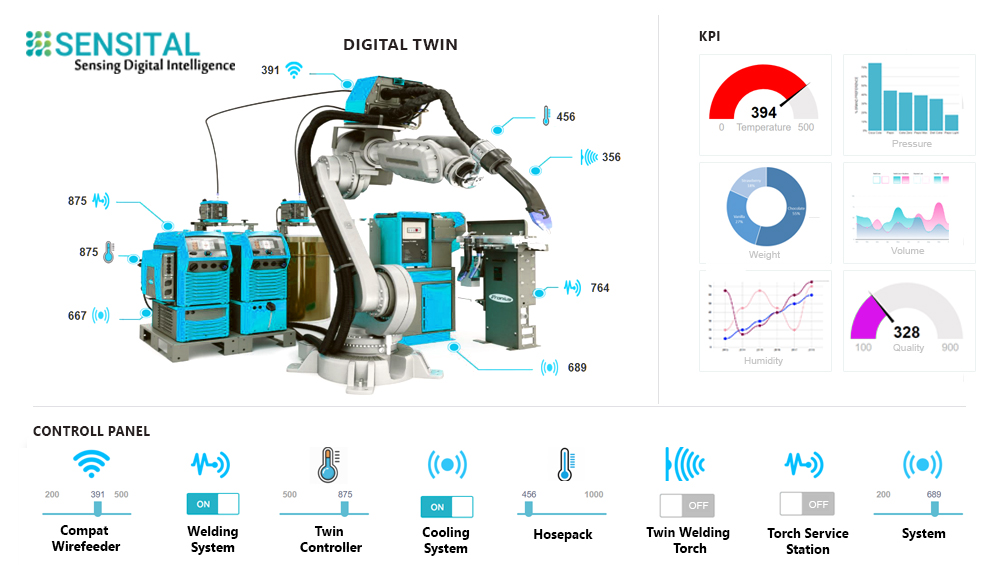
Furthermore, with its out-of-the-box integration capabilities, iBOTics can take this contextualized information and feed AI models for predicting time to failure of an asset, integrate with an enterprise IT system to initiate a maintenance request and also help forecast longer term capital expense budget.
Finally, with its bidirectional integration capabilities, Sensital can also initiate a control action to help elongate the life of and improve the health of any asset.
Sensital does all of this with simple, no-code configurations, making it easy for its user to build a true Digital Twin, configure a variety of asset types, model information flow between them and modify asset configurations over time, as needed, to extract more information and value out of the connected sensors and devices and improve decisioning.
Connect with us to learn more about how Sensital can easily help you improve your operational margins through advanced asset condition monitoring with a simple three step enablement process.